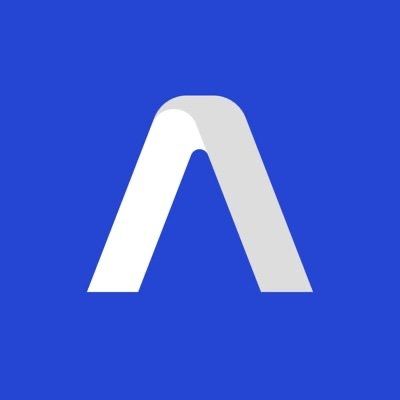
Integration: AssemblyAI
Use AssemblyAI transcription, summarization and speaker diarization models with Haystack
Table of Contents
Introduction
You can use AssemblyAI trancriptions in your Haystack 2.0 pipelines with the AssemblyAITranscriber.
With this integration, you can perform speech recognition, speaker diarization and summarization.
More info about AssemblyAI:
Installation
pip install assemblyai-haystack
Usage
The AssemblyAITranscriber
allows to perform some speech-to-text processes using the AssemblyAI API and loads the transcribed text into documents. To use this component, you should pass your ASSEMBLYAI_API_KEY
as an argument.
Based on the passed arguments, the results of the transcription, summarization and speaker diarization are returned in separate document lists:
transcription
summarization
speaker_labels
Transcription
Leverage the power of AssemblyAITranscriber
to effortlessly transcribe your audio files. By default, it outputs a single Document
object. However, for more tailored content preprocessing, you can use DocumentSplitter
.
Following example showcases an indexing pipeline that incorporates AssemblyAITranscriber
, DocumentSplitter
, and SentenceTransformersDocumentEmbedder
to preprocess audio content and store it efficiently with dense embeddings in an InMemoryDocumentStore
:
from haystack.components.writers import DocumentWriter
from haystack.components.preprocessors import DocumentSplitter
from haystack.components.embedders import SentenceTransformersDocumentEmbedder
from haystack import Pipeline
from haystack.document_stores.in_memory import InMemoryDocumentStore
from assemblyai_haystack.transcriber import AssemblyAITranscriber
document_store = InMemoryDocumentStore()
transcriber = AssemblyAITranscriber(api_key=assemblyai_api_key)
document_splitter = DocumentSplitter(
split_by = "word",
split_length = 150,
split_overlap = 50
)
document_writer = DocumentWriter(document_store)
document_embedder = SentenceTransformersDocumentEmbedder()
preprocessing_pipeline = Pipeline()
preprocessing_pipeline.add_component(instance=transcriber, name="transcriber")
preprocessing_pipeline.add_component(instance=document_splitter, name="document_splitter")
preprocessing_pipeline.add_component(instance=document_embedder, name="document_embedder")
preprocessing_pipeline.add_component(instance=document_writer, name="document_writer")
preprocessing_pipeline.connect("transcriber.transcription", "document_splitter")
preprocessing_pipeline.connect("document_splitter", "document_embedder")
preprocessing_pipeline.connect("document_embedder", "document_writer")
file_path = "https://github.com/AssemblyAI-Examples/audio-examples/raw/main/20230607_me_canadian_wildfires.mp3"
preprocessing_pipeline.run(
{
"transcriber": { "file_path": file_path}
}
)
The expected output should indicate that 9 documents are written to the document store:
{'document_writer': {'documents_written': 9}}
Note: Calling preprocessing_pipeline.run()
blocks until the transcription is finished.
The metadata of the transcription document contains the transcription ID and url of the uploaded audio file.
# {'transcript_id': ' 73089e32-...-4ae9-97a4-eca7fe20a8b1',
# 'audio_url': 'https://storage.googleapis.com/aai-docs-samples/nbc.mp3',
# }
Summarization
You can perform summarization with AssemblyAITranscriber
by setting "summarization": True
. When activated, AssemblyAITranscriber
provides both a transcription
object and a summarization
output.
The example below illustrates a generative QA pipeline that seamlessly integrates AssemblyAITranscriber
and OpenAIGenerator
. This pipeline generates answers based on the given question and the summarized transcription:
from haystack import Pipeline
from haystack.utils import Secret
from haystack.components.retrievers.in_memory import InMemoryEmbeddingRetriever
from haystack.components.builders.prompt_builder import PromptBuilder
from haystack.components.generators import OpenAIGenerator
from assemblyai_haystack.transcriber import AssemblyAITranscriber
template = """
Given the following information, answer the question.
Context:
{{summary[0].content}}
Question: {{ question }}
"""
summary_qa = Pipeline()
summary_qa.add_component("transcriber", AssemblyAITranscriber(api_key=assemblyai_api_key))
summary_qa.add_component("prompt_builder", PromptBuilder(template=template))
summary_qa.add_component("llm", OpenAIGenerator(api_key=Secret.from_token("YOUR_OPENAI_API_KEY"), model="gpt-3.5-turbo"))
summary_qa.connect("transcriber.summarization", "prompt_builder.summary")
summary_qa.connect("prompt_builder", "llm")
question="What are the air quality warnings?"
summary_qa.run({
"transcriber": {"summarization": True, "file_path": "https://github.com/AssemblyAI-Examples/audio-examples/raw/main/20230607_me_canadian_wildfires.mp3"},
"prompt_builder": {"question": question},
})
Speaker Diarization
Facilitate speaker diarization effortlessly by including the "speaker_labels": True
argument when using AssemblyAITranscriber
. This setting ensures that AssemblyAITranscriber
outputs a Document
object, containing a list of utterances. Each utterance represents an uninterrupted segment of speech from a specific speaker, and the associated speaker information is kept in the meta
field of the document.
Explore the example below to see how to index speaker diarization information and run a query pipeline with filters, allowing you to retrieve the speech text specifically from speaker A:
from haystack.document_stores.in_memory import InMemoryDocumentStore
from haystack import Pipeline
from haystack.utils import Secret
from haystack.components.retrievers.in_memory import InMemoryBM25Retriever
from haystack.components.builders.prompt_builder import PromptBuilder
from haystack.components.generators import OpenAIGenerator
from assemblyai_haystack.transcriber import AssemblyAITranscriber
## Write utterances into InMemoryDocumentStore
document_store = InMemoryDocumentStore()
file_path = "https://github.com/AssemblyAI-Examples/audio-examples/raw/main/20230607_me_canadian_wildfires.mp3"
transcriber = AssemblyAITranscriber(api_key=assemblyai_api_key)
result = transcriber.run(file_path=file_path, speaker_labels=True)
document_store.write_documents(result["speaker_labels"])
## Build a generative QA pipeline
template = """
Answer the question, based on the content in the documents. If you can't answer based on the documents, say so.
Context:
{% for document in documents %}
{{ document.content }}
{% endfor %}
Question: {{ question }}
"""
pipe = Pipeline()
pipe.add_component("retriever", InMemoryBM25Retriever(document_store=document_store, top_k=3))
pipe.add_component("prompt_builder", PromptBuilder(template=template))
pipe.add_component("llm", OpenAIGenerator(api_key=Secret.from_token("YOUR_OPENAI_API_KEY"), model="gpt-3.5-turbo"))
pipe.connect("retriever", "prompt_builder.documents")
pipe.connect("prompt_builder", "llm")
## Run the pipeline and only include the speech text from speaker A
question = "Who is more affected by wildfires?"
pipe.run({
"prompt_builder": {"question": question},
"retriever": {
"query": question,
"filters": {
"operator": "AND",
"conditions": [{"field": "meta.speaker", "operator": "==", "value": "A"}]
}
}})
Since this filtering only returns the text where person A was the speaker, it can’t find any relevant results. Run the same pipeline for speaker B information to get results.
{'llm': {'replies': ['The documents do not provide explicit information on who is more affected by wildfires.'],
'meta': [{'model': 'gpt-3.5-turbo-0613',
'index': 0,
'finish_reason': 'stop',
'usage': {'completion_tokens': 15,
'prompt_tokens': 177,
'total_tokens': 192}}]}}